Livio Fenga, Ph.D., a researcher with the Italian National Institute of Statistics, has published two papers using a statistical tool developed by Fordham professor of economics H. D. “Rick” Vinod, Ph.D. Fenga used to the tool to create data-rich projections on the future progression of COVID-19 in Italy.
The tool, known as the maximum entropy bootstrap (MEB), was made available by Vinod as an open-source computer package in 2009. Fenga’s first 14-page paper used MEB while studying various regions of Italy to estimate a “confidence interval” for the count of infected people. A confidence interval is an intuitive prediction based on hard data, said Vinod.
Using Data to Predict with Confidence
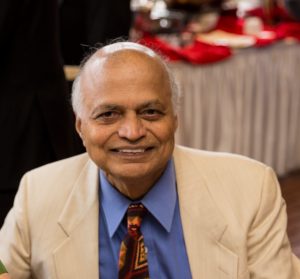
Rick Vinod
Snowfall forecasting could be used as an example of a confidence interval. When a forecaster estimates two inches of snowfall at a particular location, that two-inch prediction is called a “point estimate.” The point estimate is based on years’ worth of data that compose the forecasting models, which could be a summary of 100 scenarios considering wind velocity, temperature, and humidity.
Those two inches representing the point estimate are something easily understood by the public, much easier than the meteorologist disseminating 100 scenarios that include variables of wind, temperature, and humidity.
“That is common when the weather forecaster says the temperature will be 60 degrees, they won’t say a range,” said Vinod. “That’s how they talk because the general public doesn’t understand all the variables and they get confused.”
Such is the case when political leaders use data points to explain complex modeling to the general public amidst the current crisis. Regardless, the richer the data, the better the confidence interval, said Vinod.
“If you want to decide when to open up the economy amidst the COVID-19 crisis, for that you need to know the best- and the worst-case scenarios to create a plausible scenario range,” he said. “That should be studied with confidence intervals, like those used in Fenga’s study, not just with point estimates—not with one value but with several values.”
While continuing to use the snowfall prediction to explain the complexity of his MEB method, Vinod rhetorically asked, “What if the meteorologist has only 30 scenarios instead of 100?” That case scenario would use the “traditional bootstrap” method. If only 30 observations of snowfall numbers are available, how does one construct 100 snow scenarios?
Roughly speaking, step one would be to write the 30 snowfall numbers on a deck of 30 cards. Step two would be to pick one card at a time, write the snowfall number down, replace the card back into the deck, shuffle and repeat 100 times. A 90% confidence interval is then constructed by focusing on the middle-most 90 snowfall numbers.
Prof. Vinod’s method takes that traditional bootstrap process literally seven steps further with the “maximum entropy bootstrap” computer algorithm. It constructs confidence intervals by “shuffling short time series while preserving their unique up-and-down history over time,” he said.
Implications for Coronavirus Pandemic
The method has been especially helpful during the COVID-19 crisis when the up-and-down variables contain important information that will need to be analyzed over time. This includes a range of estimates that include an overall number of deaths for the nation, number of those tested in the nation, numbers infected, number of hospital beds, number of ICU beds, number of deaths by region, number of tests by region—and other ever-moving variables in nations greatly affected by the virus.
In Fenga’s second 19-page paper he applies MEB confidence intervals for ICU hospitalizations. He argues that MEB alone overcomes data limitations that will best help guide policy over time.
“Professor Vinod’s MEB theory is a great tool which I use quite often,” Fenga said.
Testing and Treatment Are Critical
Yet for all the innovative work produced in the two Italian studies, without random testing of the general population big national predictions are simply not possible, Vinod said.
“We need to test a representative sample of all Americans, not just those showing symptoms; that would give you a better picture of the ravages of the virus and better forecasts for what to do, but that requires test availability,” said Vinod.
Dire on-the-ground reality continues to usurp any coordinated effort at conducting such research at the moment.
“Studies aren’t the only priority, we have to treat the sick, testing general public when they’re not showing symptoms would be considered a waste—and it is a waste,” he said.
He stressed that rich data sets also can come from robust collaboration. When the virus first broke out, Vinod said, the CDC wanted to monopolize the testing. With private industry playing a role, the tests have become more efficient, he said. He cited the Abbot ID NOW COVID-19 platform as a promising example.
Economists Will Remain a Valuable Resource
He said that he is pleased to see that the work of economists, often associated with financial matters, has taken center stage in the national conversation.
“It’s great to see the medical profession apply my tools, and I continue to be interested in improving other tools as well,” he said.
At the moment he is at work “generalizing the correlation coefficient,” a statistical measure of the strength of the relationship between the relative movements of two variables that has been in use since the 1890s.
He said that of the many, many things that the virus will change about society, two constants will remain: science and data will continue to matter.
“For decision-making, it’s very important that it be science based and data based—not hope based,” he said.